Where Does Artificial Intelligence (AI) Fit In the Supply Chain? Part 2: The Present
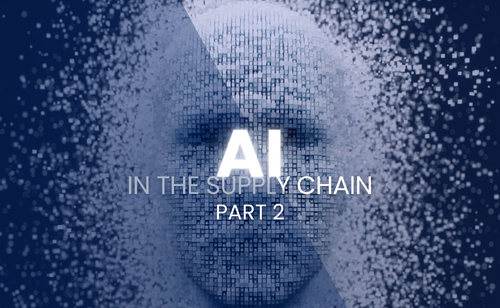
Artificial intelligence (AI) has been around for years, but more recently, the technology hit new levels of popularity when it became a household term. This is largely due to OpenAI unveiling its AI-powered chatbot, ChatGPT in November 2022. A few of the reasons ChatGPT experienced such success upon its debut was because it:
- Offers multiple payment tiers, including a freemium version
- Is easy to use
- Is trainable
- Provides valuable information to users
- Can help with everyday tasks such as writing emails, essays, and coding, as well as answering questions that users may have previously Googled
- Utilizes Generative AI
In this blog, we will be focusing on the last bullet point, Generative AI. What is it? How is it different from traditional AI that came before it? What are its strengths and weaknesses? And what role does it currently play in a B2B context, such as supply chains, ecosystem integration, trading networks, and relationship management?
Continue reading to find out.
What Is Generative AI?
Generative AI succeeded traditional AI and refers to a category of artificial intelligence systems and models that can generate new content, data, or information that is not explicitly programmed. Generative AI systems learn patterns and structures from existing data and can use that knowledge to generate new data points that conform to the identified patterns. Additionally, these systems can generate entirely new content that is similar in style or format to the data they have learned from. Generative AI can be applied to diverse applications in creative fields, data augmentation, task automation, and more.
How Does Generative AI Differ From Traditional AI?
The primary goal of generative AI is to create new data and content. It learns patterns from existing data and generates outputs that follow similar patterns. On the other hand, traditional AI aims to solve specific tasks or problems by following predefined rules and algorithms. It often relies on expert knowledge and explicit programming. Below we compare generative AI vs traditional AI.
Learning Approach
- Generative AI: Learns from data and examples, focusing on discovering patterns and relationships within the data. Common techniques include neural networks and probabilistic models.
- Traditional AI: Typically relies on rule-based systems, expert knowledge, and deterministic algorithms. It follows predefined rules and logical reasoning.
Applications
- Generative AI: Used for content generation, data augmentation, natural language processing (NLP), and recommendation systems.
- Traditional AI: Used for tasks such as data analysis, rule-based decision-making, automation of well-defined processes, and predictive analytics.
Flexibility and Creativity
- Generative AI: Known for its flexibility and creative capabilities, as it can produce a wide range of content that was not explicitly programmed.
- Traditional AI: Typically less flexible and relies on predefined rules, making them suitable for well-defined tasks but less adaptable to new challenges.
What Are Generative AI’s Capabilities?
Generative AI has expansive capabilities. Here are five notable examples:
- Data Augmentation: Generative AI generates synthetic data to improve machine learning model training. This is particularly valuable when there is limited real data available, enhancing model performance and generalization.
- Natural Language Processing (NLP): It excels in NLP tasks like text generation, translation, summarization, sentiment analysis, and chatbot interactions. It can generate coherent and contextually relevant text based on given prompts.
- Anomaly Detection: In cybersecurity and fraud detection, Generative AI identifies unusual patterns or anomalies in data, helping detect irregularities and potential threats.
- Simulation and Testing: Generative AI can simulate complex scenarios, such as autonomous vehicle testing, by generating synthetic environments and data for safe and controlled testing.
- Content Generation: Generative AI can create content across various formats, including text, images, music, and video. It can generate human-like articles, stories, art, and music compositions, and even synthesize realistic images from textual descriptions.
These capabilities underscore the versatility of generative AI, enabling its various applications in an array of contexts.
What Are Generative AI’s Shortcomings?
While a powerful and promising technology, generative AI has several shortcomings and challenges, including:
- Lack of Control: Controlling the output of generative models can be challenging. They may produce undesirable or inappropriate content, requiring strict content filtering and moderation.
- Data Dependency: Generative AI relies on vast amounts of diverse data for training, which can be costly and challenging to obtain, especially for specialized domains or languages. Data quality and availability can significantly impact model performance.
- Computational Resources: Training and deploying large-scale generative models require significant computational resources and time, making them less accessible for smaller organizations and preventing rapid development.
- Environmental Impact: Training large-scale generative models requires significant energy, contributing to the environmental footprint and raising concerns about sustainability.
- Bias, Fairness, and Ethics: Models can perpetuate biases present in their training data, leading to biased and unfair outputs. This can reinforce stereotypes, discrimination, and social inequalities. Furthermore, generative AI can be used to create deep fake content, fake news, and deceptive information, posing significant ethical concerns related to misinformation, privacy violations, and potential harm.
While these shortcomings are important to recognize, these disadvantages are being addressed and improved as generative AI technology advances.
–
Generative AI represents a pivotal advancement in the present-day field of AI, offering a wide array of practical, creative, and innovative applications. Its ability to simulate scenarios, enhance data-driven decision-making, and generate original content has limitless transformative potential across the supply chain.
While generative AI brings unprecedented opportunities for innovation and efficiency, it also poses challenges, e.g., as noted before, ethical concerns and biases. Therefore, responsible development and deployment are essential. As researchers and developers continue to refine this technology, generative AI stands as a testament to human ingenuity, offering solutions to complex problems and pushing the boundaries of what AI can achieve.
For instance, Cleo is starting to leverage generative AI and large language models in several ways that will benefit our customers. First off, we are making Cleo more efficient internally across our marketing, software/product development, and services departments. And second, we are enriching our Cleo Integration Cloud platform with powerful Gen AI capabilities, so our customers have access to the latest, most powerful information and insights. This includes product documentation, the Knowledge Base underpinning our Solution Center, and making a searchable database that’s filled with data from the thousands of integrations Cleo’s already performed, allowing our customers to discover newer, faster ways to get more done.
From Cleo’s standpoint, AI is mainly about finding innovative ways to operate smarter and faster. But as exciting as the current field of AI is, the future is even more compelling. This is especially true regarding how the future of AI will impact far-flung business ecosystems that comprise complex and fast-paced industries, such as logistics, manufacturing, wholesale distribution, and retail. To learn more about how the logistics and supply chain industries will incorporate and rely on AI technology in the years ahead, continue to Part Three in this Cleo Innovation blog series.
If you have questions about what was covered in this blog, whether it’s about AI, innovation at Cleo, API and EDI integrations in the supply chain, or Cleo’s products and services, contact us at sales@cleo.com or +1.815.282.7695. Lastly, you can explore additional educational resources through our resource library.
Related Reads:
- Artificial Intelligence (AI) in Supply Chain: The Past
- Artificial Intelligence (AI) in Supply Chain: The Future
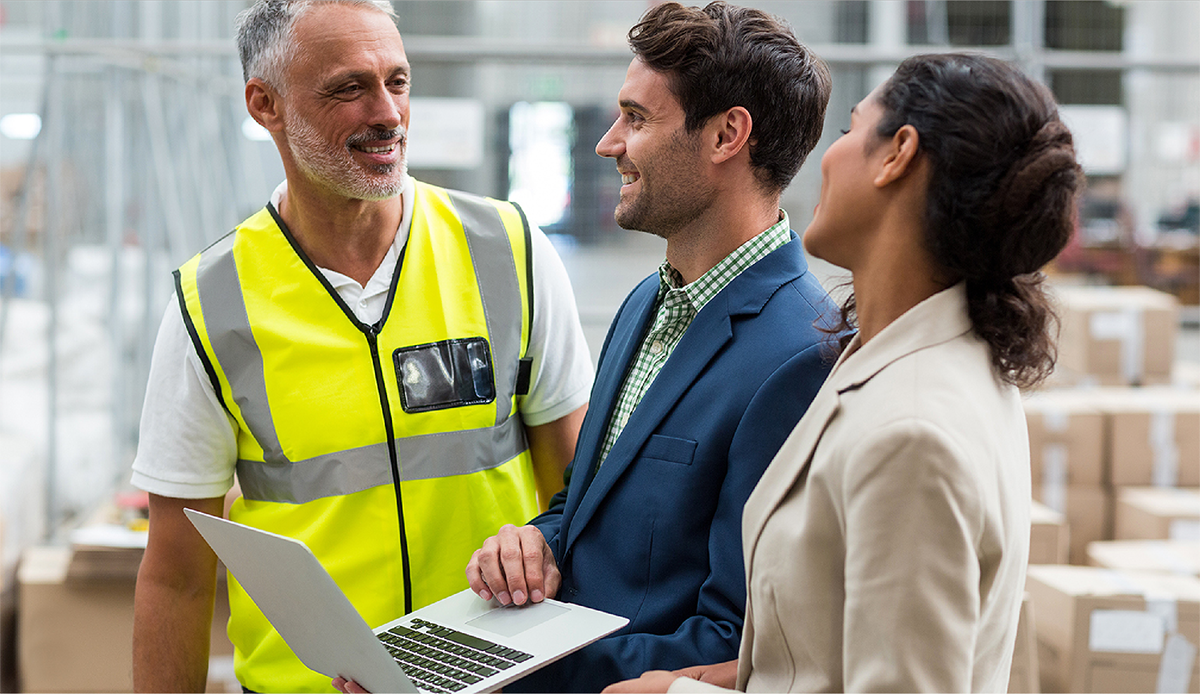
About Cleo
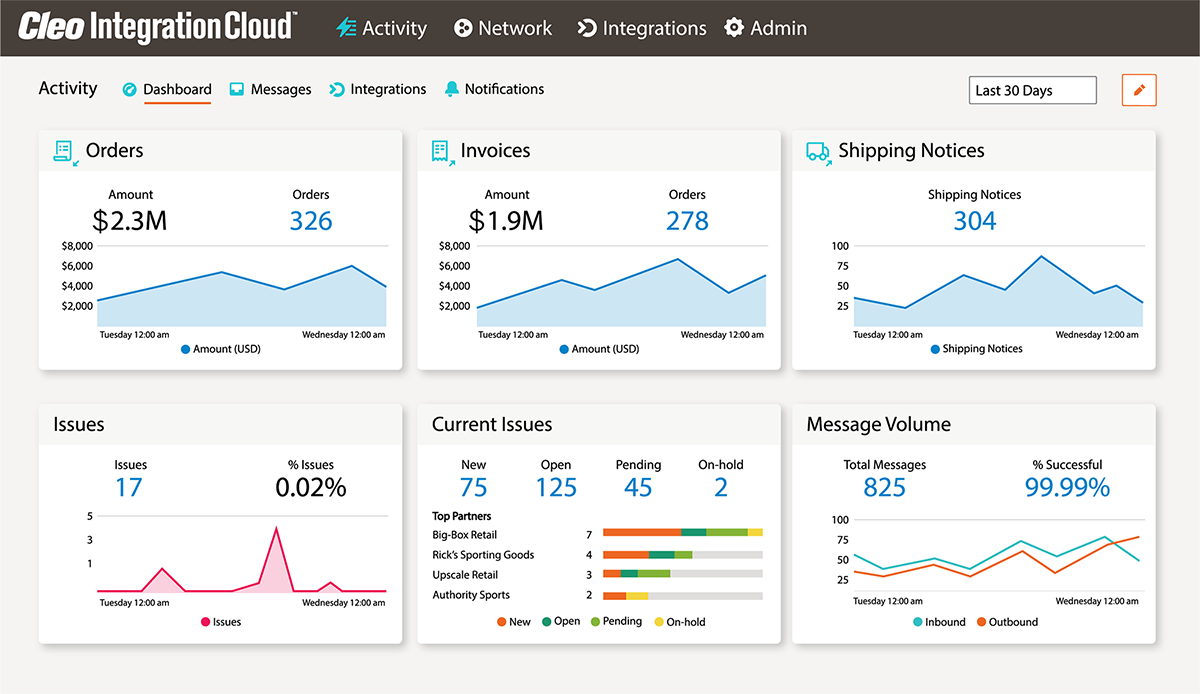
Instantly access demo videos
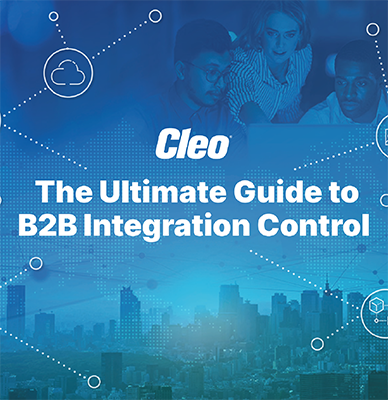
Comprehensive Guide to Gaining B2B Control
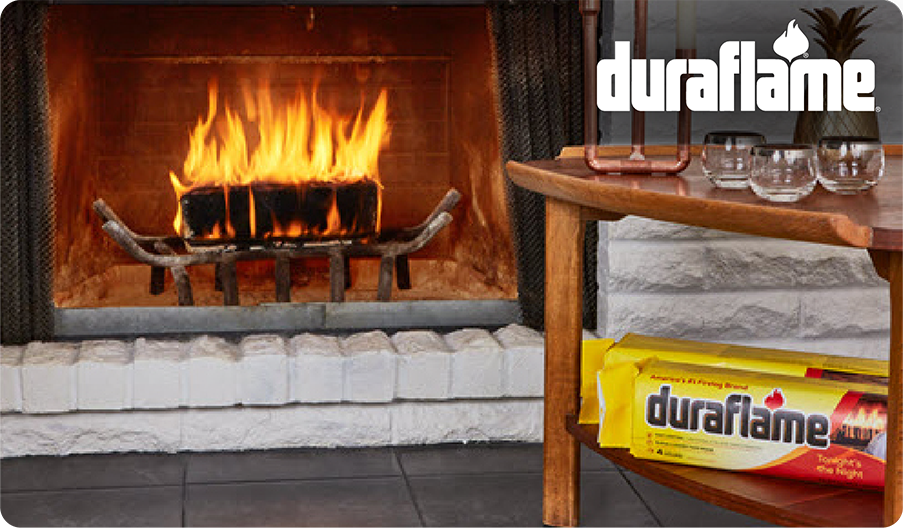
Duraflame Case Study
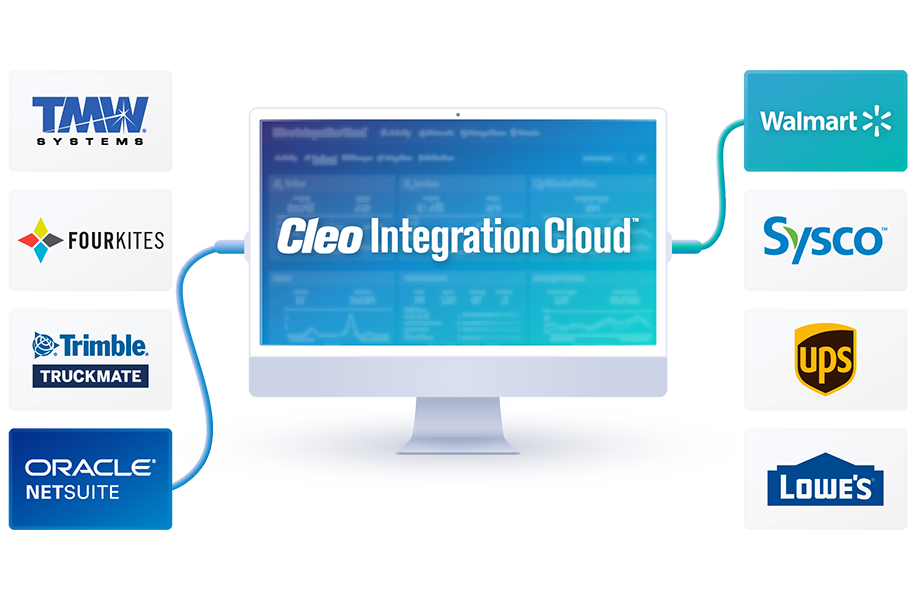